It’s no secret that AI is changing the way the world works and at the heart of it all are AI chips. These powerful little engines are what make it possible for smart tools, apps, and entire industries to run faster and think smarter.
The demand for better, faster, and more efficient AI chips has exploded over the last few years. And honestly, we owe a lot of today’s tech progress to the companies working behind the scenes to build them. They’re pushing boundaries, solving massive challenges, and setting the pace for what’s next.
In this article, we’re taking a closer look at the top AI chip makers leading the way in 2025 and why their work matters more than ever.
Top AI Chip Maker Insights
Let’s get to it.
Nvidia
You can see why Nvidia leads the AI chip market. Key stats in one punchy table:
Stat | Value |
---|---|
Founded | April 5, 1993 (Sunnyvale, CA) |
Headquarters | Santa Clara, California, U.S. |
CEO | Jensen Huang |
Employees (FY 2025) | 36,000 |
Market Cap (May 2025) | $3.24 trillion |
Revenue (FY 2025) | $130.5 billion |
Net Income (FY 2025) | $72.9 billion |
Compute & Networking Share | 89 % of total revenue |
Top AI GPU (H100) | FP16: 2 PFLOPS; 80 GB HBM3; 3 TB/s bandwidth |
Discrete GPU Market Share (Q2 2023) | 80.2 % |
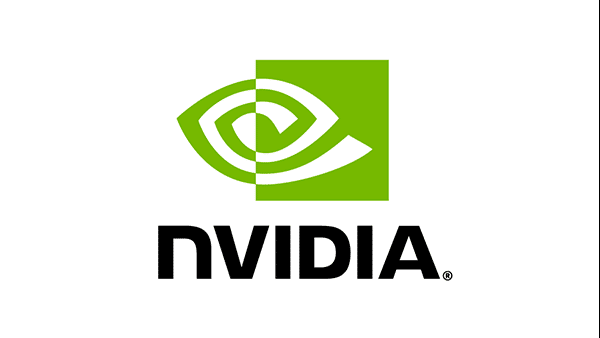
When it comes to AI chips, Nvidia is the name almost everyone knows. Founded in 1993 by Jensen Huang, Chris Malachowsky, and Curtis Priem, Nvidia started out focusing on graphics cards for gaming. But what really set them apart was their early investment in GPU (graphics processing unit) technology, which later turned out to be perfect for AI workloads.
Over the years, Nvidia shifted from being just a gaming company to a powerhouse in high-performance computing and artificial intelligence. Their CUDA platform, launched in 2006, allowed developers to use GPUs for general computing tasks, opening the door for GPUs to power AI, deep learning, and massive data processing.
Today, Nvidia controls about 80% of the AI chip market for data centers. They’ve built a strong ecosystem that combines hardware, software, and cloud services, making them the go-to choice for anyone serious about AI.
Key AI Chip(s)
I’ve pared it down to the essentials so you can compare at a glance:
Chip | Arch | Release | Tensor TF32 (TFLOPS) | Memory | Bandwidth | TDP (W) |
---|---|---|---|---|---|---|
H100 SXM | Hopper | Sep 2022 | 989 | 80 GB HBM3 | 3 350 GB/s | 700 |
A100 80 GB SXM | Ampere | May 2020 | 312 | 80 GB HBM2e | 2 039 GB/s | 400 |
L40 | Ada | Oct 2022 | 90.5 (181 w/ sparsity) | 48 GB GDDR6 ECC | 864 GB/s | 300 |
Nvidia’s most famous AI chips are the H100 Tensor Core GPUs (part of the Hopper architecture) and the newly released Blackwell GPUs (B100 and B200). These chips are built specifically to handle large language models, generative AI, and complex machine learning tasks with lightning speed and energy efficiency.
AMD
Here are the key company stats for AMD at a glance:
Stat | Value |
---|---|
Founded | May 1, 1969 |
Headquarters | Santa Clara, California, U.S. |
CEO | Lisa Su |
Employees (2024) | 28,000 |
Market Cap (May 22, 2025) | $181.70 billion |
Revenue (2024) | $25.79 billion |
Net Income (2024) | $1.641 billion |
Data Center Revenue Share (Q2 2024) | ~50 % of total sales |
Discrete GPU Market Share (Q1 2024) | 12 % |
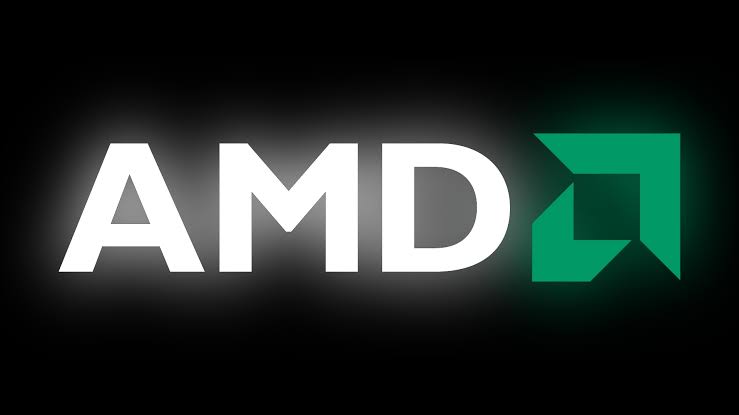
Advanced Micro Devices, better known as AMD, has been around since 1969. For a long time, AMD was seen mostly as a competitor to Intel in the CPU market. But in the last decade, thanks to smart leadership under CEO Lisa Su, AMD has completely reinvented itself, not just competing but leading in areas like high-performance computing, graphics, and now, AI.
AMD’s strategy has been to create flexible, scalable chips that can handle everything from PCs and gaming consoles to massive AI models in data centers. They’ve grown rapidly, securing major wins with tech giants like Microsoft and Meta. A big part of their success comes from designing chips that balance raw power with energy efficiency, a huge plus for AI workloads that can eat up electricity fast.
Key AI Chip(s)
Here’s a concise comparison of AMD’s leading AI accelerators:
Chip | Arch | Release | FP16 (TFLOPS) | Memory | Bandwidth | TDP (W) |
---|---|---|---|---|---|---|
Instinct MI300X | CDNA 3 | Nov 2023 | 2 614.9 (with sparsity) | 192 GB HBM3 | 5.3 TB/s | 750 |
Instinct MI250X | CDNA 2 | Nov 2021 | 383.0 | 128 GB HBM2e | 3.2 TB/s | 500 |
Instinct MI100 | CDNA | Nov 16 2020 | 184.6 | 32 GB HBM2 | 1.228 TB/s | 300 |
AMD’s flagship AI chips right now are the MI300 series, particularly the MI300X and MI300A. These chips are purpose-built for AI training and inference, and they’re already being deployed in supercomputers and cloud data centers around the world.
Intel
Here’s a crisp overview of Intel’s key company metrics, followed by a snapshot of their flagship AI accelerators:
Stat | Value |
---|---|
Founded | July 18, 1968 |
Headquarters | Santa Clara, California, U.S. |
CEO | Lip-Bu Tan |
Employees (2025) | 102,600 |
Market Cap (May 22, 2025) | $90.25 B |
Revenue (2024) | $53.1 B |
Net Income (2024) | – $18.8 B |
Data Center Revenue Share (2024) | 19.3 % |
CPU Market Share (Q1 2025) | 75.6 % |
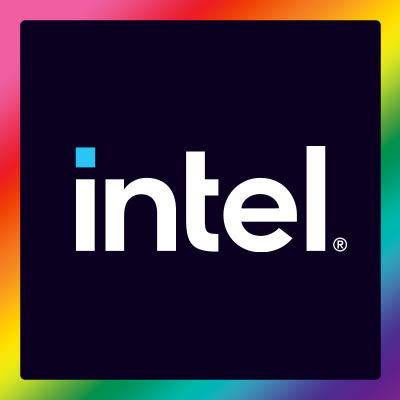
Intel is one of the original giants of the semiconductor world, founded way back in 1968. While they’ve long been known for CPUs that powered generations of computers, Intel has been aggressively pushing into AI in recent years. They’ve invested heavily in both in-house development and acquisitions (like Habana Labs and Nervana Systems) to speed up their AI efforts.
Intel’s approach is all about covering the full spectrum of AI needs, from low-power AI chips for edge devices to massive accelerators for data centers. They’ve been steadily modernizing their technology to keep up with newer players, focusing on both hardware and software solutions that make AI development more accessible and scalable.
Key AI Chip(s)
Chip | Architecture | Release Date | TFLOPS | Memory | Bandwidth | TDP |
---|---|---|---|---|---|---|
Intel Gaudi 3 | Gaudi 3 | Late Sep 2024 | 1,678 (BF16/FP8) | 128 GB HBM2e | 3.7 TB/s | 900 W |
Intel Gaudi 2 | Gaudi 2 | May 10 2022 | ~ 400 (FP16/BF16) | 96 GB HBM2e | 2.45 TB/s | 600 W |
Data Center GPU Max 1550 | Xe-HPC | Jan 10 2023 | 29.5 (FP32) | 128 GB HBM2e | 3.28 TB/s | 6 |
Intel’s big AI play right now is the Gaudi series — especially the Gaudi2 and the newly launched Gaudi3 chips. These are specifically built for training and running large AI models. Gaudi chips offer an alternative to Nvidia’s GPUs, promising strong performance at a lower total cost, which has caught the attention of a lot of cloud providers.
Amazon Web Services (AWS)
Here’s a crisp overview of AWS as a company, followed by key specs for its custom AI chips:
Stat | Value |
---|---|
Founded | Launched July 2002 (platform); EC2 & S3 in 2006 |
Headquarters | Seattle, Washington, U.S. |
CEO | Matt Garman |
Employees (Amazon total, 2024) | 1,556,000 |
Revenue (2024) | $107.6 billion |
Operating Income (2024) | $39.8 billion |
Global Cloud Market Share (2023) | 31 % |
Active Customers | Over 1 million |
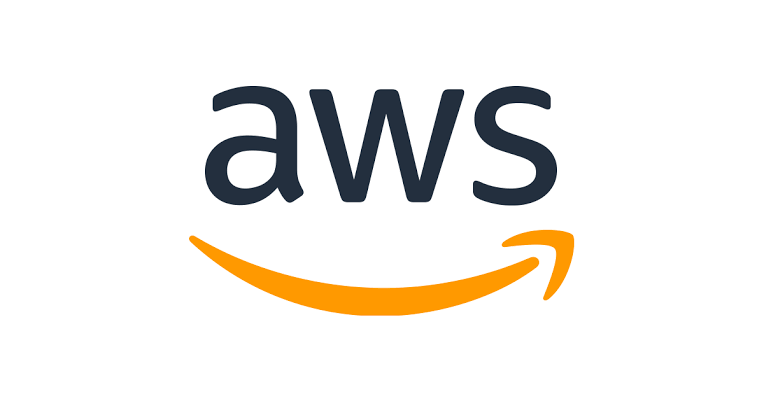
Amazon Web Services, or AWS, may be best known for its cloud computing services, but it’s also a big player in AI chip development. AWS isn’t traditionally a hardware maker like Nvidia or Intel, but they’ve made waves in the AI space with their own custom-designed chips. Their strategy is focused on providing cost-effective, scalable AI solutions through the cloud.
AWS launched its first custom AI chip, the Inferentia, in 2018. Inferentia was specifically designed to handle machine learning inference workloads, offering a more affordable and efficient solution for businesses running AI models in the cloud. Following up, AWS introduced the Trainium chip in 2021, designed for training deep learning models, providing top-tier performance for large-scale AI training.
AWS’s AI chips are unique because they are tightly integrated with their cloud services, making it easier for businesses to scale AI operations without worrying about hardware procurement. By offering these chips as part of their cloud services, AWS makes cutting-edge AI accessible to businesses of all sizes, not just those with massive budgets for hardware.
Key AI Chip(s)
Chip | Architecture | Release | Compute Throughput | Memory | Bandwidth |
---|---|---|---|---|---|
Graviton3 | ARM Neoverse V1 (64-core) | May 2022 | up to 25 % better compute vs Graviton2 | DDR5 (8 channels) | 307.2 GB/s |
Trainium2 | NeuronCore-v2 | Dec 2024 | up to 83.2 PFLOPS FP8 (sparse) | 6 TB HBM3 | 185 TB/s |
Inferentia2 | NeuronCore-v2 | May 2023 | up to 2.3 PFLOPS BF16/FP16 | 32 GB HBM | 9.8 TB/s |
Inferentia (for inference) and Trainium (for model training) are AWS’s flagship AI chips, optimized for cloud-based AI applications.
Here’s a snapshot of Google as a company, followed by the key specs of their main AI chips:
Stat | Value |
---|---|
Founded | September 4, 1998 |
Headquarters | Mountain View, California, U.S. |
CEO | Sundar Pichai |
Employees (2024) | 183 323 |
Market Cap (May 22, 2025) | $2 045.48 B |
Revenue (2024) | $350.018 B |
Net Income (2024) | $100.1 B |
Google Cloud Market Share (Q4 2024) | 12 % |
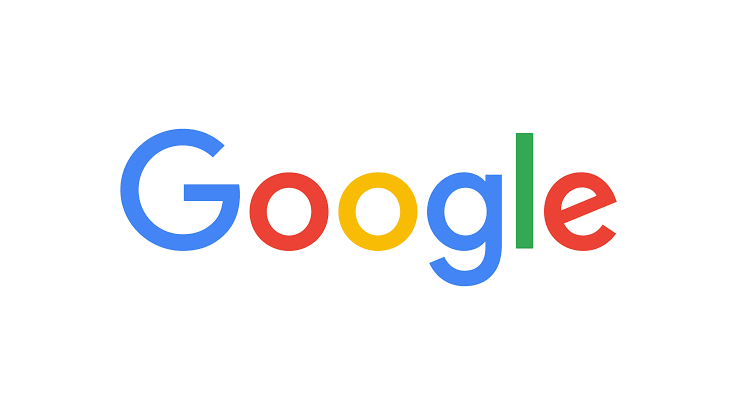
Google has long been a leader in AI, and their custom-designed hardware is one of the driving forces behind their dominance. Google’s AI journey began in 2016 with the launch of the Tensor Processing Unit (TPU), a custom chip designed specifically for accelerating machine learning tasks. Unlike many of the other AI chipmakers, Google’s focus has always been on optimizing these chips for their own use within Google Cloud, Google Search, YouTube, and other services.
TPUs are key to Google’s AI infrastructure, handling everything from training deep learning models to running inference tasks for billions of users. These chips have been used to power major advancements in AI, including Google Translate, Assistant, and Google Photos. While TPUs were originally developed in-house, Google has also made them available to third-party developers via Google Cloud, making cutting-edge AI hardware accessible to a wider audience.
Google’s push with TPUs continues to evolve, with newer generations offering faster processing speeds, lower power consumption, and enhanced efficiency. They’ve even made AI a major selling point for their cloud services, positioning Google Cloud as a go-to platform for businesses looking to leverage advanced machine learning models.
Key AI Chip(s)
Chip | Release Year | TOPS (TeraOps) | Memory | Bandwidth | TDP (W) |
---|---|---|---|---|---|
TPUv4 | 1200 GB/s | 275 WikipediaGoogle Cloud | 32 GiB HBM | 1 200 GB/s | 170 |
TPUv3 | 2018 | 123 | 32 GiB HBM | 900 GB/s | 220 |
TPUv2 | 2017 | 45 | 16 GiB HBM | 600 GB/s | 280 |
Tensor Processing Units (TPUs) are Google’s flagship AI chips, developed to accelerate both AI model training and inference tasks.
Cerebras Systems
Here’s a glance at Cerebras Systems and the wafer-scale AI engines they’ve built:
Stat | Value |
---|---|
Founded | 2015 |
Headquarters | Sunnyvale, California, U.S. |
CEO | Andrew Feldman |
Employees (2024) | 401 |
Revenue (2023) | $78.7 million |
Total Funding Raised | $720 million |
Valuation (Series F, 2021) | $4 billion |
IPO Target Valuation (2024) | $7–8 billion |
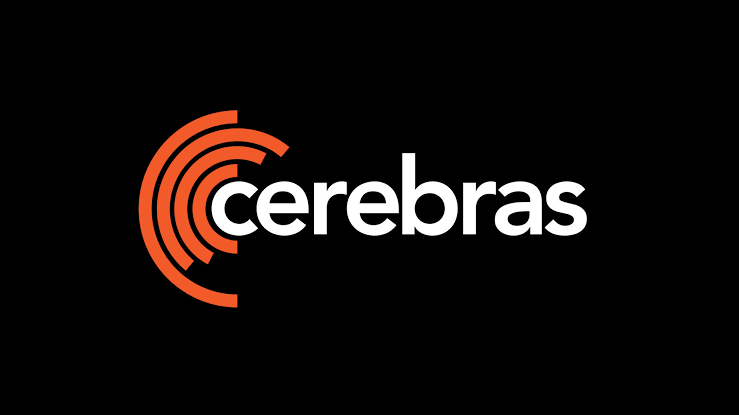
Cerebras Systems is a relatively newer player in the AI chip market, but its innovative approach has made waves. Founded in 2015 by Andrew Feldman and a group of tech veterans, Cerebras set out to create chips capable of handling the massive scale of AI workloads, particularly those needed for training deep neural networks.
What sets Cerebras apart is its focus on a single-chip supercomputer. Their flagship product, the Wafer-Scale Engine (WSE), is the world’s largest AI chip. The WSE is designed to handle entire models on a single chip, making it capable of processing huge amounts of data with unprecedented speed and efficiency. This design eliminates bottlenecks that occur when traditional AI chips (like GPUs) rely on connecting multiple chips together.
Cerebras has primarily targeted research institutions and large enterprises with complex AI needs. Their chips are used for tasks like natural language processing, medical research, and weather modeling, where massive data sets and high processing power are required.
Key AI Chip(s)
Chip | Release | Process | Cores | Transistors | On-Chip Memory | Peak AI Perf |
---|
WSE-1 | Aug 2019 | 16 nm | 400 000 | 1.2 trillion | 18 GB SRAM | — |
WSE-2 | Apr 2021 | 7 nm | 850 000 | 2.6 trillion | 40 GB SRAM | — |
WSE-3 | Mar 2024 | 5 nm | 900 000 | 4 trillion | 44 GB SRAM | 125 PFLOPS |
The Wafer-Scale Engine (WSE), now in its third generation (WSE-3), remains Cerebras’s flagship chip, revolutionizing AI training by providing unmatched scale and speed.
Qualcomm
Here’s a snapshot of Qualcomm as a company, and the key specs for its Cloud AI 100 accelerators:
Stat | Value |
---|---|
Founded | 1985 |
Headquarters | San Diego, California, U.S. |
CEO | Cristiano Amon |
Employees (2024) | 49 000 |
Revenue (2024) | $38.962 B |
Net Income (2024) | $10.142 B |
Market Cap (May 22 2025) | $161.8 B |
QCT Revenue Share (Fiscal 2024) | 85.1 % |
Smartphone AP Market Share (2023) | 39 % |
Baseband Processor Share (2023) | 50 % |
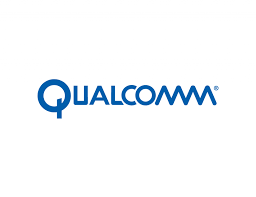
Qualcomm has long been a giant in the mobile chip industry, but in recent years, they’ve expanded into the AI space with a focus on edge AI. Founded in 1985, Qualcomm initially made its mark by developing chips for mobile phones. However, with the rise of AI, they quickly recognized the potential for integrating AI directly into mobile and IoT (Internet of Things) devices.
Qualcomm’s approach to AI is centered around making AI more accessible and efficient on smaller, lower-power devices. Their AI Engine, which powers chips like the Snapdragon 8 series (used in premium smartphones), is specifically designed to perform AI tasks like voice recognition, object detection, and real-time image processing on mobile devices without needing to rely on cloud computing.
Qualcomm’s edge AI solutions are crucial for applications in mobile, automotive, and IoT, where latency, power consumption, and real-time decision-making are critical. With their AI-driven Snapdragon chips, Qualcomm aims to push the boundaries of what’s possible on the edge, bringing AI-powered experiences to everyday devices.
Key AI Chip(s)
Chip Variant | Form Factor | INT8 (TOPS) | FP16 (TFLOPS) | AI Cores | On-Die SRAM | DRAM | TDP |
---|---|---|---|---|---|---|---|
Cloud AI 100 Standard | PCIe HHHL | 350 | 175 | 16 | 144 MB | Up to 32 GB LPDDR4x | 75 W |
Cloud AI 100 Pro | PCIe HHHL | 400 | 200 | 16 | 144 MB | Up to 32 GB LPDDR4x | 75 W |
The Snapdragon AI Engine (featured in Snapdragon 8 Gen 2) is Qualcomm’s flagship chip for mobile and edge devices, delivering powerful AI capabilities while optimizing power consumption.
IBM
Stat | Value |
---|---|
Founded | June 16, 1911 |
Headquarters | 1 Orchard Road, Armonk, New York, U.S. |
CEO | Arvind Krishna |
Employees (2024) | 270 300 |
Revenue (2024) | $62.73 billion |
Net Income (2024) | $6.023 billion |
Market Cap (May 23, 2025) | $234.96 billion |
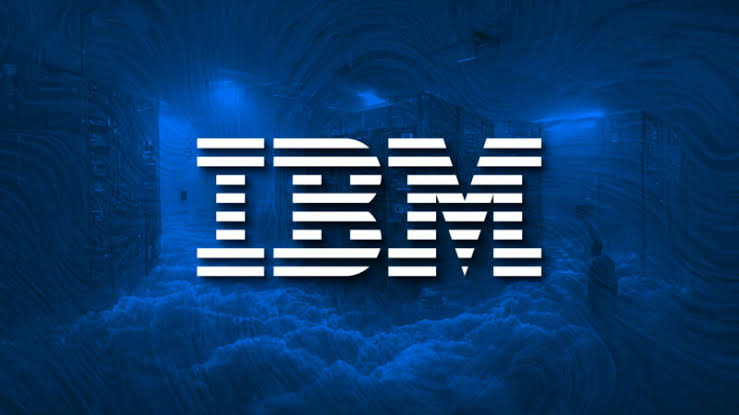
IBM, a tech giant since 1911, has been a significant player in AI for decades. While it might not always make the same headlines as companies like Nvidia or Google, IBM’s contributions to AI, particularly in enterprise solutions, are immense. IBM’s AI efforts are largely built around its Watson platform, which uses machine learning and natural language processing to help businesses automate complex tasks.
What makes IBM’s approach unique is their focus on AI at scale for industries like healthcare, finance, and manufacturing. They’ve designed AI chips and systems to power data centers, and their development of PowerAI integrates machine learning tools directly into their IBM Power Systems. These systems are optimized for AI workloads and provide companies with the computational power needed for large-scale AI training and deployment.
Although IBM’s AI chips are not as well-known in the consumer space as some others, they are incredibly important in enterprise applications, particularly in fields where AI adoption is helping solve complex challenges like drug discovery or predictive maintenance.
Key AI Chip(s)
Chip | Architecture | Release | Process | Cores & Threads | Clock (GHz) | AI Accelerator | Cache (L2 per core; L3/L4) |
---|
Telum | z/Architecture | 2021 | 7 nm | 8 cores | > 5.0 | Neural Network Processing Assists (NNPA) | 32 MB L2; 256 MB L3; 2 GB L4 |
Power10 | Power ISA v3.1 | 2021 | 7 nm | Up to 15 cores, SMT8 | 3.5–4.0 | Matrix Math Accelerator (MMA) engines | 2 MB L2 per core; 120 MB L3 |
IBM’s POWER9 processors, used in conjunction with Watson AI, offer exceptional performance for enterprise-level AI applications. More recently, IBM has been focusing on integrating AI into their IBM Power Systems for seamless, scalable solutions.
Broadcom
Here’s a crisp overview of Broadcom Inc., followed by specs for two of its leading AI-infrastructure chips.
Stat | Value |
---|---|
Founded | 1961 |
Headquarters | Stanford Research Park, Palo Alto, California, U.S. |
CEO | Hock Tan |
Employees (2024) | 37 000 |
Revenue (2024) | US $51.57 B |
Operating Income (2024) | US $13.46 B |
Net Income (2024) | US $5.895 B |
Market Cap (May 2025) | US $1.08 T |
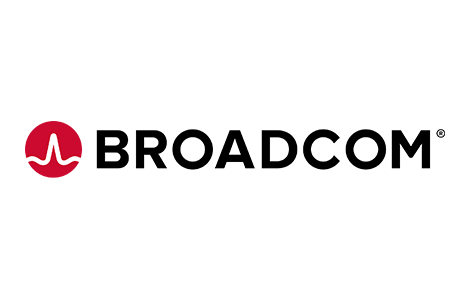
Broadcom, founded in 1991, is a semiconductor company that might not be the first name you think of when it comes to AI chips, but they’re deeply embedded in the infrastructure that supports AI technologies. Broadcom’s focus has traditionally been on networking, wireless communication, and broadband, but they’ve made significant strides in developing chips for AI and machine learning applications.
Broadcom’s AI push is mainly through its networking solutions, providing hardware for AI-driven data centers and high-performance computing systems. Their chips support the infrastructure that powers AI applications, including storage, memory, and interconnect solutions that ensure smooth communication between AI workloads. Broadcom has also invested heavily in AI accelerators and networking technology, which are crucial for training AI models quickly and efficiently across distributed systems.
By focusing on the foundational elements of AI hardware, Broadcom plays a critical role in enabling the high-speed data transfer and storage that AI systems rely on. They continue to develop products that enhance AI performance in areas like networking and cloud computing.
Key AI Chip(s)
Chip | Release | Throughput | Ports | Memory | Process |
---|---|---|---|---|---|
Tomahawk 5 | Aug 2022 | 51.2 Tbps | 64 × 800 GbE | – | – |
Jericho2 (BCM88690) | Mar 2018 | 4.8 Tbps | 12 × 400 GbE | 8 GB HBM2 Gen2 | 16 nm |
Broadcom’s Tomahawk and Trident series are leading networking chips that help accelerate AI data transfer within data centers, ensuring high-performance computing and smooth AI operation.
Tenstorrent
Here’s a snapshot of Tenstorrent as a company, followed by specs of its top AI chips:
Stat | Value |
---|---|
Founded | 2016 (Toronto, Canada) |
Headquarters | Santa Clara, California, U.S. (offices in Toronto & Austin) |
CEO | Jim Keller |
Employees (2024) | 140 |
Total Funding | ~$1 billion |
Latest Valuation | $2.6 billion (post-money, Dec 2024) |
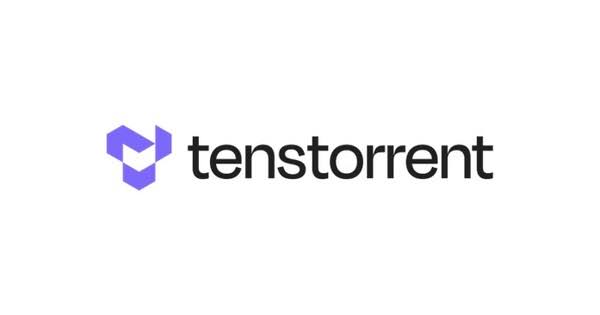
Founded in 2016 by Jim Keller, a renowned chip designer known for his work at companies like AMD and Tesla, Tenstorrent is an innovative startup focused on building next-gen AI chips. The company has rapidly gained attention due to its forward-thinking approach to AI hardware and its goal to provide highly efficient processors for machine learning and deep learning applications.
Tenstorrent’s flagship offering is the Grayshift processor, which is designed to accelerate AI model training with an emphasis on scalability, flexibility, and performance. The company’s chips stand out by integrating both scalar and vector processing units, making them versatile enough for a variety of AI tasks. Tenstorrent is also known for developing a distributed architecture that helps speed up training for large-scale deep learning models.
The company’s vision is to help businesses and researchers scale their AI workloads without facing the limitations posed by existing hardware solutions. Despite being relatively new, Tenstorrent has already attracted significant investment and partnerships, making them one to watch in the evolving AI chip landscape.
Key AI Chip(s)
Chip | Architecture | Release | Tensix Cores | SRAM | Memory | Bandwidth | TFLOPS (FP8) | TFLOPS (FP16) |
---|---|---|---|---|---|---|---|---|
Grayskull e150 | Tensix | Announced 2020 | 120 | 120 MB | 8 GB LPDDR4 | 118.4 GB/s | 332 | 83 |
Wormhole n300 | Tensix (2×) | July 2024 | 128 (64 per ASIC) | 192 MB (96 per ASIC) | 24 GB GDDR6 | 576 GB/s | 466 | 131 |
Grayshift processors are Tenstorrent’s primary focus, designed to optimize AI training at scale with an architecture that supports both scalar and vector processing.
Groq
Stat | Value |
---|---|
Founded | 2016 |
Headquarters | Mountain View, California, U.S. |
CEO | Jonathan Ross |
Employees (2023) | 250 |
Revenue (2023) | US $3.2 million |
Net Income (2023) | US $−88 million |
Series C Funding (Apr 2021) | US $300 million |
Series D Funding (Aug 2024) | US $640 million |
Valuation (Aug 2024) | US $2.8 billion |
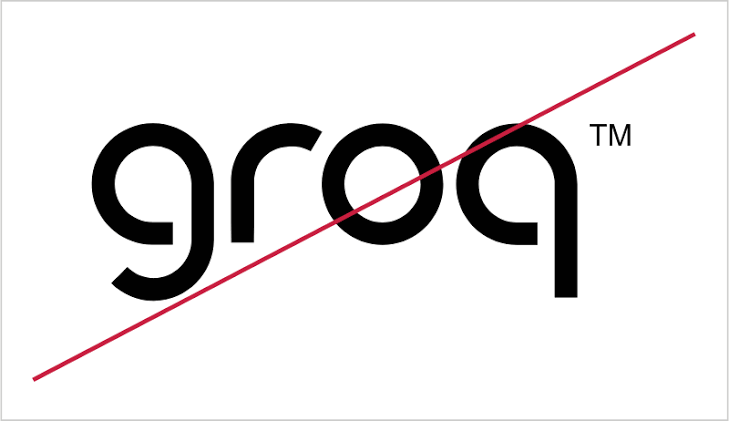
Founded in 2016, Groq is another fresh and ambitious player in the AI chip market. What sets Groq apart is its unique approach to AI acceleration. The company was founded by Jonathan Ross, a former Google engineer who worked on the TensorFlow machine learning platform. Groq’s mission is to simplify AI chip design and deliver an architecture that can handle the massive workloads of AI models with low latency and high throughput.
Groq’s Tensor Streaming Processor (TSP) is their standout product, built from the ground up to accelerate AI computations at the chip level. Unlike traditional GPUs or other accelerators, Groq’s TSP is designed for extreme parallelism, allowing it to handle a wide variety of AI tasks, from training models to inference. The TSP can deliver high performance for both image recognition and language processing at an impressive speed.
In a short period, Groq has attracted attention from major AI research groups and tech companies, positioning itself as a highly efficient and scalable alternative to other traditional AI accelerators.
Key AI Chip(s)
Chip | Architecture | Process & Size | Clock | Peak Compute | On-chip SRAM | Memory Bandwidth |
---|---|---|---|---|---|---|
LPU v1 (TSP) | Tensor Streaming Processor (LPU) | 14 nm; 25 × 29 mm | 900 MHz | 250 TFLOPS FP16; 1 PetaOPS | ~200 MB | 80 TB/s |
GroqCard™ Accelerator | LPU-based PCIe card | 14 nm; PCIe form factor | 900 MHz | 188 TFLOPS FP16; 750 TOPS INT8 | 230 MB | 80 TB/s |
The Tensor Streaming Processor (TSP) is Groq’s primary chip, designed to provide high throughput and low-latency performance for large-scale AI applications.
Iluvatar Core X
Here’s a concise look at Iluvatar CoreX and its two flagship AI GPUs:
Stat | Value |
---|---|
Founded | 2015 |
Headquarters | Shanghai, China |
Founder | Li Yunpeng |
CEO | Diao Shijing |
Employees (2024) | 300 |
Total Funding | $334 million |
Valuation (2024) | >$1 billion |
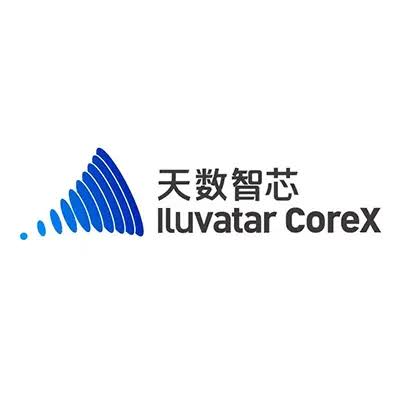
Iluvatar is a relatively newer entrant in the AI chip industry but is quickly gaining attention for its innovative designs and high-performance AI accelerators. The company was founded with the goal of developing chips that are specifically optimized for AI and deep learning applications, addressing the unique challenges that these workloads present.
Their Core X chip is at the forefront of Iluvatar’s offerings. It is built to provide powerful AI processing while maintaining energy efficiency — a crucial factor for scaling AI operations, especially in edge computing and mobile applications. The Core X is designed for both training and inference, with a focus on high-throughput capabilities and low-latency performance.
What sets the Core X apart is its unique hybrid architecture, combining elements of both traditional CPUs and specialized AI cores, allowing it to handle a wide range of tasks efficiently. Whether it’s powering AI-driven robotics, autonomous vehicles, or real-time data analytics, the Core X aims to provide a versatile solution for next-generation AI systems.
Despite its newer status, Iluvatar has built partnerships with several startups and research institutions, showing strong potential for growth in the AI hardware space.
Key AI Chip(s)
Chip | Process | Precision Performance | Memory | Bandwidth | Use Case |
---|---|---|---|---|---|
TianGai-100 | 7 nm | FP16: 147 TFLOPS | 32 GB HBM2 | – | Training |
ZhiKai-100 | 7 nm | FP32: 24 TFLOPS; FP16: 96 TFLOPS; INT8: 384 TOPS | – | 800 GB/s | Inference |
Core X, a versatile AI chip optimized for both training and inference with a hybrid architecture aimed at real-time processing.
Final Thoughts
So, while we may not always think about the tech behind AI, it’s these companies that make it all possible. From Nvidia’s high-performance chips to AWS and Google pushing the limits of cloud computing, the advancements happening in the AI chip space are shaping everything we do. Companies like Cerebras and Tenstorrent are shaking things up with new chip designs, and Qualcomm and Broadcom are bringing AI power right to your pocket.
It’s great to see how all these players are working together to make AI more powerful, efficient, and accessible. And as AI keeps growing, it’s clear that these companies will be at the heart of it all, helping AI do things we’re just beginning to imagine.
Did we miss your favorite AI chip maker? Drop a comment below, and we might just do a part 2!